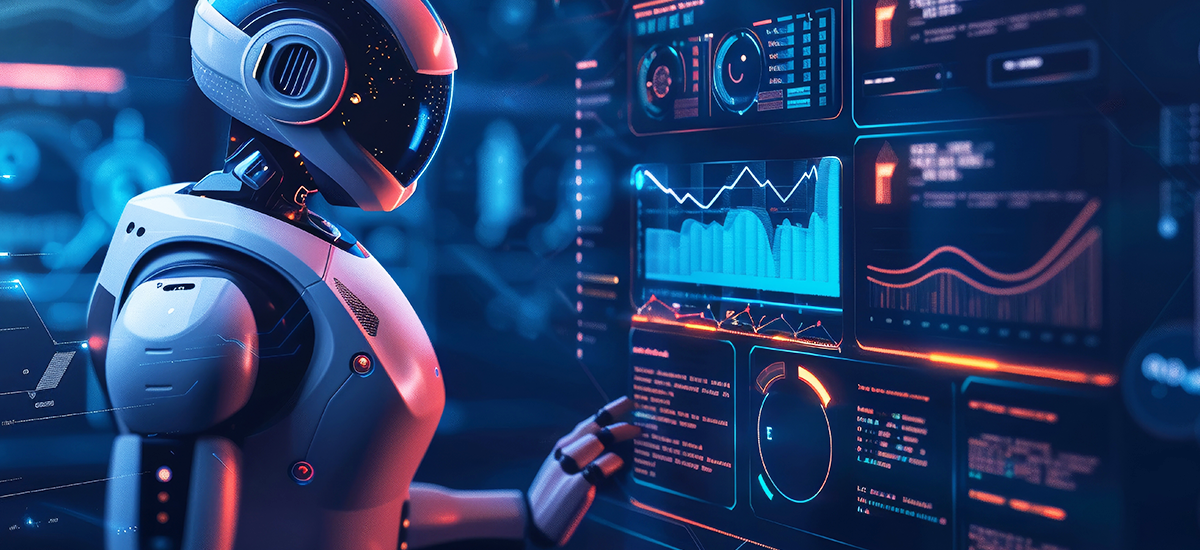
Listen to this blog
Introduction
Generative AI (GenAI) is changing the Insurance landscape by enhancing underwriting accuracy, streamlining claims processing, and improving customer service. A survey reveals that 76% of Insurance companies in the U.S. have incorporated generative AI into at least one business function, with claims processing, customer service, and distribution being the primary areas of adoption. However, scaling AI models from a pilot project to full-scale production presents unique challenges, particularly around compliance, data quality, and integrating AI into legacy systems. In this blog, we’ll explore how Insurance companies can overcome these hurdles to ensure successful GenAI implementation.
1. Turning AI pilots into policyholders: Overcoming the gap between testing and full-scale implementation
Challenge:
In the Insurance industry, GenAI pilots often demonstrate success in isolated areas like fraud detection or claims automation. However, scaling these solutions to handle the complexities of real-world data, such as regulatory compliance, customer diversity, and real-time decision-making, can pose significant technical challenges.
Solution:
Design GenAI pilots with scalability in mind, ensuring that they can handle large volumes of claims and data from multiple sources. Use cloud platforms like AWS or Google Cloud to scale infrastructure dynamically. Implement compliance-ready systems from the beginning, ensuring that AI models align with regulations like GDPR and HIPAA.
2. Data and compliance first: Addressing governance issues in Insurance AI
Challenge:
Inconsistent, incomplete, or poor-quality data is a significant problem in Insurance. AI models rely heavily on accurate and structured data from multiple sources, such as claims reports, medical records, and policyholder data. Mismanaged data can lead to incorrect underwriting decisions or faulty fraud detection.
Solution:
Develop a robust data governance framework that centralizes and standardizes data. Implement AI-powered data cleaning tools to ensure data quality. Use secure cloud storage (e.g., Snowflake or AWS) to manage large datasets efficiently and maintain compliance with data privacy laws.
3. Cost-benefit balancing act: Overcoming the complexity and cost barriers to AI in Insurance
Challenge:
The costs of implementing and maintaining GenAI solutions can be prohibitive for Insurance companies, especially when working with multiple, fragmented platforms that increase operational complexity.
Solution:
Centralize AI workloads on unified platforms that can scale with the business. Leverage cloud solutions, such as AWS or Microsoft Azure, to consolidate data storage and analytics, improving system efficiency while reducing costs. Use cloud-native AI tools for continuous performance monitoring and cost optimization.
4. Making AI accessible for underwriters and claims adjusters: Democratizing Insurance AI tools
Challenge:
Insurance professionals (underwriters, claims agents) may lack the technical expertise to work directly with AI systems, leading to a communication gap between IT teams and business units.
Solution:
Invest in AI tools that are accessible to non-technical users, providing intuitive interfaces for underwriting, claims processing, and customer service teams. Cloud-based solutions, like AWS SageMaker and Microsoft Azure, facilitate easy interaction between IT and business teams, allowing AI-driven insights to be applied directly to everyday operations.
5. Ethics and regulations in Insurance AI: Overcoming governance challenges in a sensitive industry
Challenge:
Insurance companies are required to meet stringent regulatory requirements (e.g., GDPR, HIPAA). Ensuring data privacy, fairness, and transparency in AI decision-making is crucial to avoid legal or reputational risks.
Solution:
Implement AI governance frameworks that include strict data security protocols and transparent decision-making processes. Leverage encryption and anonymization techniques to ensure customer data is protected. Conduct regular audits for bias and fairness to maintain regulatory compliance.
6. Seamless AI integration: Adapting GenAI into the Insurance claim and underwriting workflows
Challenge:
Insurance companies often rely on legacy systems for claims processing, underwriting, and customer management. Integrating GenAI models with these existing workflows can be complex.
Solution:
Use APIs and microservices to integrate AI solutions with legacy systems. Implement middleware platforms that can bridge gaps between old systems and new AI-driven tools. Ensure that GenAI solutions are modular, allowing seamless integration into the existing IT ecosystem without disrupting daily operations.
7. AI that grows with you: Continuous monitoring and scaling for the Insurance sector
Challenge:
AI models in Insurance need to adapt continuously as new data comes in, such as updated claims or changing customer behavior. Without ongoing maintenance, models can become outdated, leading to less accurate predictions.
Solution:
Set up automated systems for continuous model retraining and real-time performance monitoring. Use cloud-based GenAI platforms to scale models quickly and efficiently as new data becomes available. Track performance metrics such as claims accuracy and fraud detection rate, to evaluate AI effectiveness.
8. Adapting to change: Organizational preparedness for GenAI in Insurance
Challenge:
GenAI adoption can be hindered by organizational resistance, especially if there is a lack of understanding of its potential. Misalignment between IT and business teams can further slow down the implementation process.
Solution:
Invest in GenAI training programs to increase literacy across teams, focusing on how it can improve daily workflows. Ensure alignment between IT and business departments, establishing, clear objectives and fostering collaboration. Implement a change management plan that highlights the benefits of GenAI for operational efficiency and customer satisfaction.
9. Tracking AI investment: Managing costs and measuring the ROI of GenAI in Insurance
Challenge:
Scaling GenAI in Insurance often requires significant financial investment, and quantifying the return on investment (ROI) can be difficult.
Solution:
Define clear success metrics during the pilot phase, such as reduced claim processing times or improved underwriting accuracy. Use cloud-based pricing models like pay-as-you-go, to optimize resource allocation and reduce costs. Continuously measure the financial impact of GenAI on operational efficiency and customer satisfaction to validate ROI.
Conclusion
For Insurance companies, successful GenAI adoption hinges on robust data governance, seamless integration with legacy systems, and a focus on improving customer experience through automation and personalized services. With the growing demand for faster claims processing and enhanced customer support, GenAI can be a game-changer in reducing costs and boosting efficiency. Start by evaluating your current processes—take the first step toward GenAI-driven transformation now.