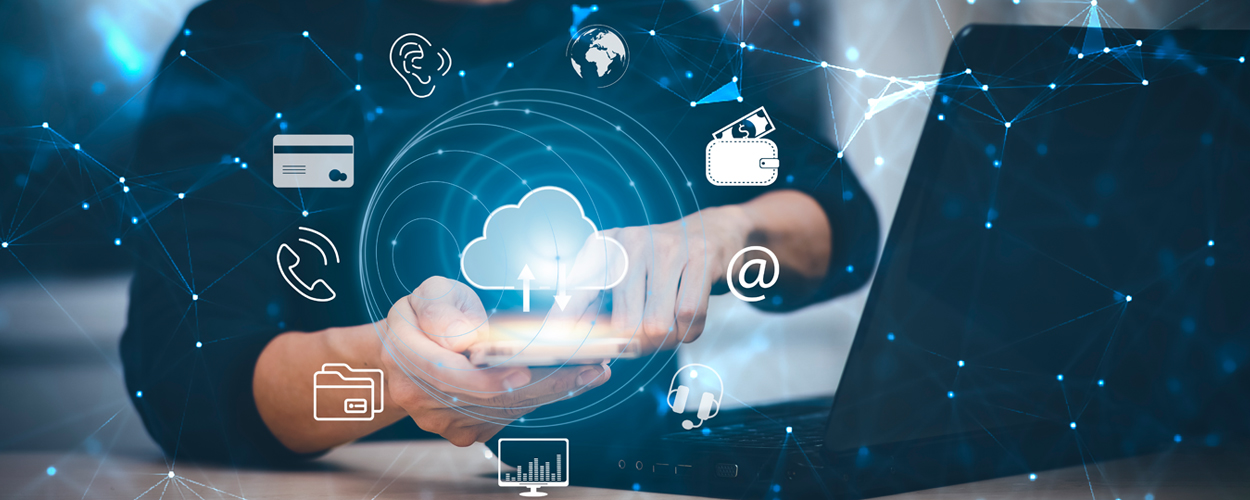
Today’s IT teams thrive in dynamic environments leveraging agile methodologies, continuous integration/continuous delivery, and distributed services across the multi-cloud realm. With a sprawl of microservices and containers, and increased release velocity, it becomes impossible for the human eye to cope with the complexity that defines these environments. The increasingly digital landscape of today stipulates a seamless user experience, demanding IT and DevOps teams to resolve issues before they escalate. More than simply knowing what problem has occurred, they also need to know why it has happened – and that too in real time.
Traditional monitoring tools and processes struggle to keep up with the explosive amounts of data being generated in these environments and simply can’t deliver the required level of observability. True observability is achieved by collecting all the granular telemetry data, applying intelligence to contextualize it, and making informed decisions from it. Combining observability with AIOps, i.e., applying artificial intelligence (AI) and data science to IT operations, makes incident response less stressful. It exponentially improves MTTR, reduces alert noise, and increases confidence of deploying features rapidly.
Why is AI so important for modern observability?
Observability is at its best when implemented with AIOps as it enables automation and intelligent view into the entire infrastructure. Let’s take a look at a couple of key reasons why AI is vital to ensuring true observability.
Supports SRE functions in proactive issue detection and action
AI is an all-important necessity when it comes to proactive detection and action. It enables SRE teams to do their jobs effectively. In addition to quickly getting to the root cause of problems, AI and ML can allow prediction of possible issues long before they even escalate. Downtime of business-critical applications can cost millions of dollars, reputational damage, and loss of customers which can have long-term repercussions. Additionally, this can result in loss to internal productivity, penalties and legal issues.
Enhances DevOps efficacy
Organizations adopt DevOps model to maintain proper alignment between Dev and Ops teams. AIOps delivers an essential tool in their arsenal to help them work smarter and faster by automating data analysis and routine DevOps operations. By providing the Ops teams with full visibility into the developers’ work and delivering a comprehensive view of the IT environment to the Dev teams, AIOps helps streamline collaboration between them. This, in turn, helps the CI/CD processes run with zero downtime and helps in faster application development. This explains the prediction by Gartner where they highlighted in a recent report that by 2022, AIOps platforms that monitor, support, and deploy applications will help DevOps teams increase their delivery cadence by 20%.
Reduces monitoring noise
ITOps tools deal with thousands of events across on-premise and cloud environments, which gives rise to monitoring noise. AIOps leverages technologies like pattern recognition, vent correlation, and anomaly detection to present just the mission-critical alerts that need to be addressed.
How to make the most of observability and AIOps?
Gartner predicts that the number of business leaders relying on AIOps for automated insights will increase by 10x by the year 2024. IT teams can uncover a wealth of information out of all the data once they figure out its path and how it is being used as a final insight.
AIOps use cases
Some common use cases of AIOps are as follows:
- Finding similar incidents to accelerate issue resolution
- Identification of problems based on anomalies in normal behavior
- Forecasting value of a particular metric to improve operational readiness
- Grouping alerts, events or logs based on text descriptions or symptoms
- Grouping of similar alerts based on attributes
- Determining application or server health based on consolidated telemetry data
- Classification of incidents using natural language processing or any external service
- Identification of correlated time series metrics or symptoms for faster root cause identification
- Assisting cybersecurity functions to enhance the speed, visibility, and intelligence of data security and threat detection
- Processing results with machine learning to trigger automated system responses
- Identification of outliers in configurations and applications to aid in cohort analysis
Explore the transformative potential of observability and AIOps
Building highly observable systems requires a thorough understanding of applications and services. Observability and AIOps provide the context and awareness required for automated issue remediation and zero-disruption operations, while allowing DevOps teams to focus on the customer experience.
This transformative impact of AIOps is catching the eye of IT leaders as they get increasingly enthusiastic about the promise of applying AI to IT operations. Gartner predicts that large enterprise exclusive use of AIOps and digital experience monitoring tools to monitor applications and infrastructure will rise from 5% in 2018 to 30% in 2023.
Join our upcoming live webinar “Unravelling Container Observability” where we will discuss in detail on how to augment observability with intelligence and how the combination of observability and AIOps maintains uptime and peak efficiency of business-critical applications. Our panel will also highlight how to overcome challenges associated with observability to ensure highly agile, secure and scalable systems for application development. Reserve your spot today.