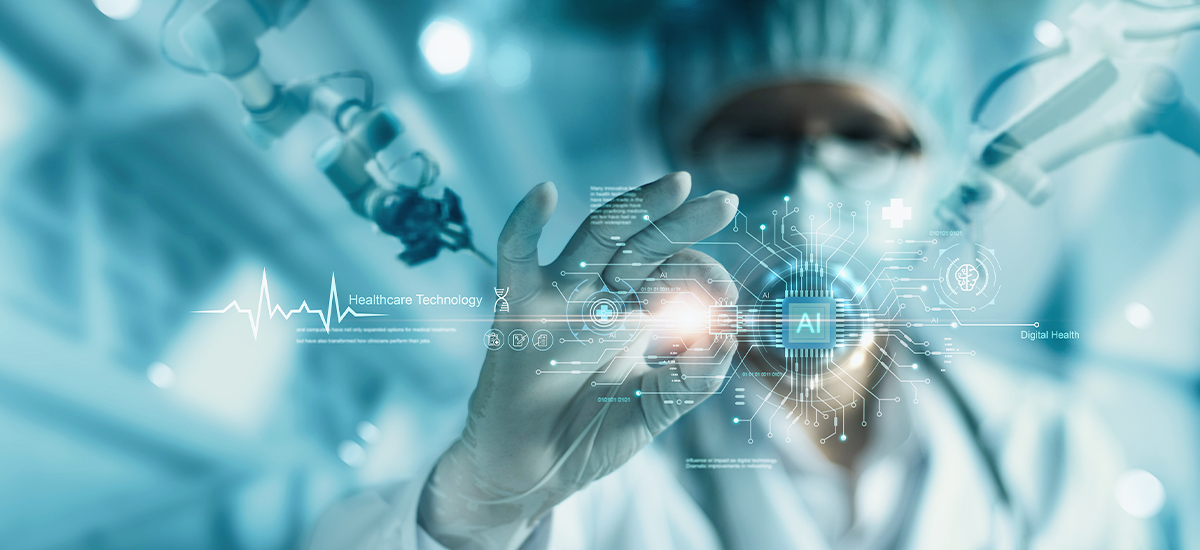
Listen to this blog
Introduction
Generative AI (GenAI) holds transformative potential in the Life sciences, Pharmaceutical, and Healthcare industries. From accelerating drug discovery and improving diagnostics to enhancing personalized treatment plans, GenAI is poised to revolutionize these sectors. In fact, McKinsey estimates that GenAI could unlock between $60 billion to $110 billion annually for the Pharmaceutical industry alone by enhancing drug discovery processes and speeding up clinical trials. However, scaling GenAI projects from successful pilots to full-scale production presents unique challenges, including data governance, integration complexities, regulatory compliance, and cost management. In this blog, we’ll explore how these retail leaders can overcome these hurdles to fully unlock the power of GenAI.
1. From pilot to patient: Bridging the gap between AI trials and real-world Healthcare impact
Challenge:
In Healthcare and Pharma, pilots may demonstrate promising results in isolated areas such as drug research or patient monitoring. However, moving from a small-scale pilot to full-scale production introduces several complexities. These include managing large datasets, aligning AI models with evolving clinical practices, meeting stringent regulatory requirements (such as HIPAA, FDA guidelines), and addressing infrastructure scalability challenges for real-time applications.
Solution:
Design GenAI pilots with scalability in mind, integrating cloud infrastructure that can handle large-scale data and comply with industry regulations. Ensure GenAI solutions are adaptable to the fast-changing healthcare environment by using cloud platforms like AWS, Azure, or Google Cloud to scale infrastructure and automate the deployment process. Additionally, involve regulatory and clinical experts early in the design to ensure alignment with industry standards and protocols.
2. Ensuring quality and compliance: Addressing data challenges in Healthcare AI
Challenge:
Data quality is critical in the Healthcare and Pharmaceutical industries. Inaccurate or incomplete medical records, drug efficacy data, and patient demographics can lead to biased models and potentially harmful outcomes. Additionally, fragmented data from disparate systems, like electronic health records (EHR), clinical trials, and lab reports, complicates AI model training and validation.
Solution:
Implement a strong data governance framework that centralizes and standardizes data. Use AI-driven data cleansing techniques to ensure accurate and consistent datasets. Leverage cloud platforms, like Snowflake or AWS, for centralized data management and ensure compliance with healthcare data privacy regulations (e.g., HIPAA). Consider using blockchain technology for immutable data records, particularly in clinical trials, to maintain data integrity.
3. Unlocking Healthcare potential: Managing the complexity and cost of GenAI in the medical field
Challenge:
AI model deployment in Life sciences and Healthcare can be costly, given the need for large-scale data processing and high-performance computing. Fragmented AI platforms can lead to inefficiencies, resulting in longer time-to-market for drug development, higher operational costs, and potential data latency issues when models are scaled.
Solution:
Adopt unified AI platforms that centralize Data and AI workflows, reducing complexity and operational costs. Cloud-based solutions such as AWS SageMaker or Microsoft Azure AI, can help streamline model development, training, and deployment, providing a pay-as-you-go model that reduces upfront investment. This approach ensures resources are optimized and can be scaled on demand without excessive capital expenditure.
4. AI for all: Democratizing access to GenAI in medicine and research
Challenge:
In the Healthcare and Pharmaceutical sectors, many stakeholders—including clinicians, researchers, and administrative staff—may not have the technical expertise to use AI tools directly. This creates a barrier to widespread AI adoption, hindering collaboration between technical teams and business teams in areas like patient care or clinical trial management.
Solution:
Focus on making AI tools user-friendly and accessible to non-technical users. Platforms like Microsoft Power BI, AWS, and Google Cloud offer intuitive interfaces that allow researchers, clinicians, and business teams to access AI insights without needing deep technical knowledge. Provide comprehensive training and workshops to increase AI literacy across departments, ensuring that AI tools are used effectively across all stages of drug development, clinical trials, and patient care.
5. Staying ethical and compliant: Regulatory and governance hurdles for Healthcare AI
Challenge:
The Healthcare and Life sciences industries are heavily regulated, with strict data privacy requirements and ethical concerns surrounding GenAI applications, such as biased diagnostic tools or incorrect drug recommendations. Managing these regulatory challenges while ensuring the fairness and transparency of AI models can be complex, especially in critical applications like clinical decision support and personalized medicine.
Solution:
Develop and implement robust governance frameworks that include encryption, access control, and data security measures to ensure compliance with regulations such as HIPAA, GDPR, and FDA guidelines. Leverage explainability frameworks to ensure that AI models provide interpretable outputs, which is essential in medical applications. Regularly audit AI systems for bias and fairness, particularly in areas like patient diagnosis, treatment suggestions, and drug efficacy predictions, ensuring that AI does not unintentionally perpetuate healthcare disparities.
6. Integrating GenAI into Healthcare: Connecting AI solutions to clinical and research workflows
Challenge:
Integrating GenAI models into existing healthcare workflows can be difficult. Healthcare organizations typically rely on complex, legacy systems (e.g., Electronic Health Records, clinical data management systems, and ERP software). Integrating these models into day-to-day clinical or administrative operations requires significant effort, both from a technical and organizational perspective.
Solution:
Ensure that AI models can be integrated seamlessly with existing healthcare systems using APIs and microservices. This ensures minimal disruption to healthcare workflows. Utilize integration tools like FHIR (Fast Healthcare Interoperability Resources) standards to bridge the gap between AI systems and clinical data sources. Involve key stakeholders—such as clinical staff, IT teams, and regulatory bodies—early in the design process to ensure smooth integration and adoption.
7. Continuous care for AI models: Monitoring and scaling GenAI in Healthcare
Challenge:
In Life sciences and Healthcare, AI models need constant updates to remain accurate and relevant, especially as new research findings, patient outcomes, and regulatory changes emerge. Without continuous monitoring and model retraining, AI systems risk becoming outdated, leading to inaccurate clinical predictions or suboptimal drug discovery outcomes.
Solution:
Implement continuous monitoring systems that track AI performance over time, focusing on key metrics like diagnostic accuracy, patient outcomes, and drug efficacy. Set up automated model retraining pipelines that update models as new data becomes available. Use agile methodologies to ensure that GenAI solutions remain flexible and scalable in response to the rapidly evolving nature of healthcare and medical research.
8. Preparing Healthcare for AI transformation: Readiness and change management in the medical field
Challenge:
Resistance to GenAI adoption is common in healthcare organizations, especially when staff members feel that AI might replace their roles or disrupt established workflows. Additionally, the complexity of AI models and the lack of AI literacy can lead to slow adoption across different teams, from clinicians to administrative staff.
Solution:
Invest in training programs to improve AI literacy across the organization. Focus on educating healthcare professionals about how GenAI can augment their decision-making, rather than replacing it. Promote a culture of innovation and collaboration by aligning IT and clinical teams around common AI goals. Involve leadership in advocating for AI-driven solutions to foster organizational readiness for adoption.
9. Healthcare’s ROI on AI: Measuring cost efficiency and impact
Challenge:
In Healthcare and Pharma, scaling GenAI requires a substantial investment in technology, talent, and infrastructure. Measuring the ROI of AI in these sectors can be challenging, especially when the benefits—such as improved patient outcomes, faster drug development, or operational efficiencies—are long-term or indirect.
Solution:
Clearly define success metrics during the pilot phase, such as reduced diagnosis times, increased drug discovery success rates, or improved patient satisfaction. Use cost-effective cloud solutions like AWS or Microsoft Azure, which offer flexible pricing models (e.g., pay-per-use) to help manage AI operational costs. Continuously track performance metrics and adjust strategies to demonstrate ROI, justifying further investment in AI.
Conclusion
In the Life sciences and Healthcare industries, GenAI offers immense opportunities, but its success lies in aligning GenAI with stringent regulatory standards, ensuring data integrity, and fostering collaboration across clinical and IT teams. With the potential to accelerate drug discovery and enhance patient care, the key is to drive innovation while maintaining the highest standards of compliance. It’s time to capitalize on GenAI’s potential—begin your journey towards adopting scalable GenAI-driven Healthcare solutions today.