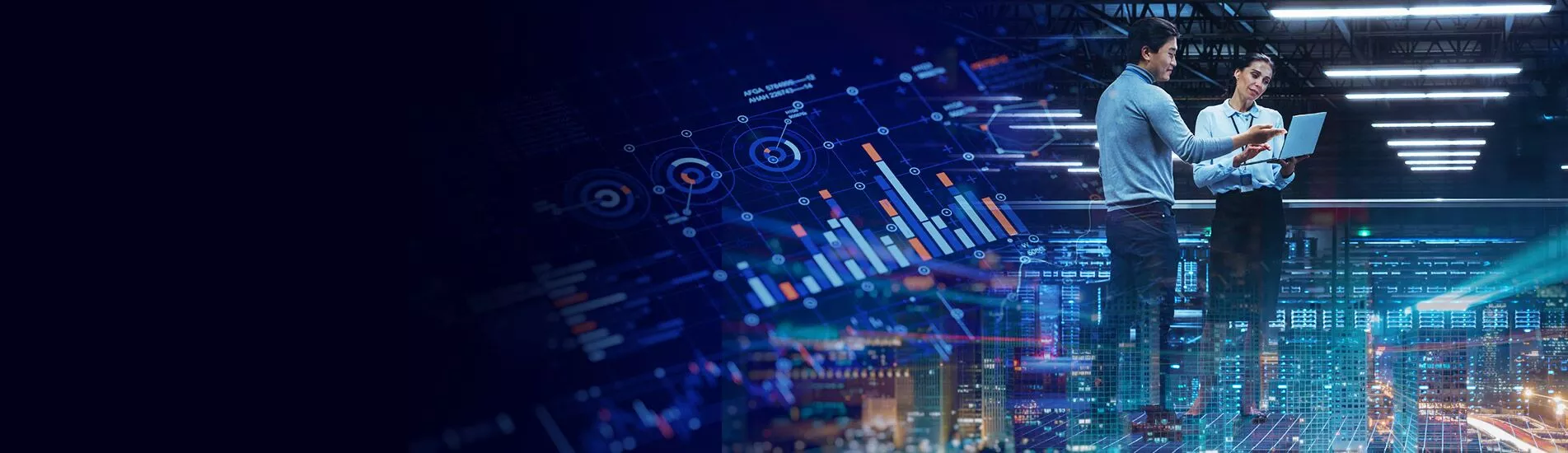
Listen to this blog
In the data-driven world, modern data technologies are changing how businesses operate. Automated decision-making, simplifying large data chunks in minutes, and gaining actionable insights in real time is just the tip of the iceberg. With encompassing business benefits, it is of no surprise why many progressive companies hold a preference for modern data technologies.
Traditional data warehouses and data lakes are no longer viable, failing to meet the high business demands of challenging times. This is why enterprises today are increasingly keen to embrace cutting-edge technologies, making sure they are aligned with a standardized data view at a corporate level and avoid common failure points standing the test of time. What could be better than a Data Lakehouse for such an approach?
What is Data Lakehouse?
A Data Lakehouse strategically integrates management capabilities and tools from data warehouses with flexible unstructured data storage from data lakes to create a broader solution. Users benefit from the best of both worlds through the assimilation of these tools.
With a Data Lakehouse, users get a data management platform that brings together the unstructured records from the data lake with the processed and structured records from the data warehouse. This unified modern data platform seamlessly accelerates data processes by removing silos between traditional data repositories. Moreover, Data Lakehouse offers a contextual semantic layer that ensures less administration, improved governance, and enhanced functionality while keeping costs low. Users can leverage AI/ML-powered data management and business intelligence (BI) tools that enable seamless data cleansing and schema enforcement while ensuring complete control over data security.
Figure 1: A Comparative View of Modern Data Warehouse and Data Lakehouse Methodologies.
Figure 2: Progression of Data Warehousing (Image sourced from Databricks)
Data Lake Challenges and Warehouse Volatility
Data Lake promises to be a better alternative to a data warehouse. However, this progressive technology comes with its own set of limitations, such as:
Reprocessing Missing Data
Missing or corrupt data during data processing is one of the biggest enterprise challenges. Failure to access the database can lead to huge losses in terms of time, money, and deletion of the compromised data.
Ensuring Data Adequacy
To ensure data reliability, having a sound data validation mechanism is crucial. Failing to meet this core essential can cause corruption of data pipelines at critical periods.
Additional Overheads for Batch and Streaming Data
To ensure every form of the data stream, including batch and streaming data, is effectively managed, your Data Lake must have the desired data processing capacity. Engineers often turn to a practice referred to as “Lambda Architecture” that serves the purpose. However, this mechanism comes along with its own set of extensive requirements. One of the major issues is that it requires a pair of codes for batch and stream processing. These additional activities add to the maintenance and development overheads of the entire data platform.
Consistency with Regular Upgrades, Integration, and Disposal
One of the elementary components of any business success is maintaining data consistency. Failure to instill such a mechanism and ensure seamless compliance with regulations (CCPA and GDPR) undoubtfully becomes a nuisance for companies striving to run smoothly in the long run.
Poorly Managed Data Lakes
Due to the absence of essential characteristics such as data governance and quality assurance, data lakes fail to make the most of their potential. When not structured well, documented with poor standards, or managed incompetently, these data lakes turn into data swamps.
Data Cleansing Challenges
Another crucial element when dealing with data processing is data cleansing. However, when it comes to data lakes, there is high variability in data formats and data types, making the task extremely challenging. In this case, data cleansing for schema information requiring a rational and logical approach pertains to only a certain extent.
Progression to Latest Innovation: Data Lakehouse
Changing with the ever-evolving industries demands a continuous shift to the latest innovations in core business elements, and data management is no exception. It is the amalgamation of data lake and data warehouse, infusing the best of both into one solution. It enables smooth data management via open system architecture and empowers businesses with data lake storage flexibility and cutting-edge features comparable to the data warehouse. Enabling businesses to save time and resources via role-based access to data repositories, it also helps to seamlessly accelerate business processes without investing in numerous systems.
The Framework of Data Lakehouse: A Comprehensive View
Figure 3: The framework and process flow of the cutting-edge Data Lakehouse.
Data Lakehouse: Key Features
By combining the value addition of both the data warehouse and the data lake, the Data Lakehouse framework augments the preceding data lakes, resulting in various process enhancements, including:
Comprehensive Transactional Support:
Enables ACID (atomic, consistent, isolated, durable) transactions, it simplifies processes through faster developments, seamless inserts and deletes, and schema validation, ensuring end-to-end process standardization.
Reliability with Schema Validation and Governance:
Enables end-to-end data reliability and governance via effective schema or database validation. This unified data platform allows business users to modify or change the data anytime without compromising its data accuracy and availability.
Faster BI Support and Maintenance:
Data Lakehouse enables business users to make prompt data queries by leveraging Business Intelligence (BI) tools. As a result, this speeds up BI support and increases efficiency by eliminating extraneous tasks such as creating unique data copies in the reporting layer.
Supports Multiple Data Formats:
Whether it is structured, raw, or partially structured, the Data Lakehouse framework supports nearly all data formats. It allows business users to seamlessly record, enhance, and evaluate data irrespective of its data type (image, visual, document, sound).
Comprehensive Streaming:
This framework holds all the necessary tools and features to enable real-time data streaming. Its comprehensive mechanism excludes the need to invest in other avenues while simplifying end-to-end streaming through a unified data platform.
AI/ML Capabilities:
Machine learning has become more prevalent than ever owing to the massive growth of business data over the years. Customer-centric companies are shifting to new tools and methodologies and enhancing their data management capabilities. However, infusing AI/ML capabilities is crucial to sustaining resilient data infrastructures. Data Lakehouse simplifies this process. It helps data engineers swiftly leapfrog through the data versioning – enabling seamless development of AI/ML models.
Affordable Than Conventional Versions:
One of the promising elements of Data Lakehouse is that it is more cost-effective than conventional methods. Its sophisticated framework enables continuous process improvement for businesses while helping users maintain end-to-end data integrity.
Resources to Develop a Lakehouse
– Google BigQuery (BQ)
One of the exclusively managed data warehousing applications that Google Cloud Platform (GCP) offers is Google BigQuery (BQ). This application paves the path for many businesses to seamlessly employ the Data Lakehouse framework and efficiently transition to data lakes.
– Apache Drill
In order to build resilient Data Lakehouse frameworks, it is essential to start by finding a high-performance querying engine such as Apache Drill. Apache Drill supports an extensive set of file formats (JSON, CSV, PSV), empowering businesses to combine multiple queries into a single one. However, the process of dataset cleansing takes longer to complete. As soon as the process gets done, this low latency distributed query engine meticulously simplifies the querying process, helping you efficiently create your Data Lakehouse.
– Delta Lake
Another elemental open-source storage architecture that helps to develop the Data Lakehouse framework is Delta Lake by Databricks. Owing to its exclusive features, this platform-agnostic storage layer ensures the reliability and resilience of data lakes. Additionally, Delta Lake supports faster data ingestion, enables smooth access to and compliance with ACID transactions, helps manage metadata, and provides consistency by combining batch and streaming data pipelines empowering businesses with time travel capabilities.
Lakehouse Architecture is Here to Stay
Traditional data architectures will undoubtedly succumb in the face of the new age of data warehousing enhancements. One of these emerging solutions, Data Lakehouse, is cautiously designed to elevate enterprise-wide performance. This modern data platform enables users with open and direct access to data, helping them effectively process data by harnessing the power of business intelligence (BI) and machine learning (ML) capabilities.
This open data management framework empowers businesses to seamlessly amplify their business performance and productivity by leveraging the collective benefits of data lakes and data warehouses, packaging it with their self-service data management competencies.
Want to learn more about how modern Data Lakehouse can help your business grow? Get in touch with us.