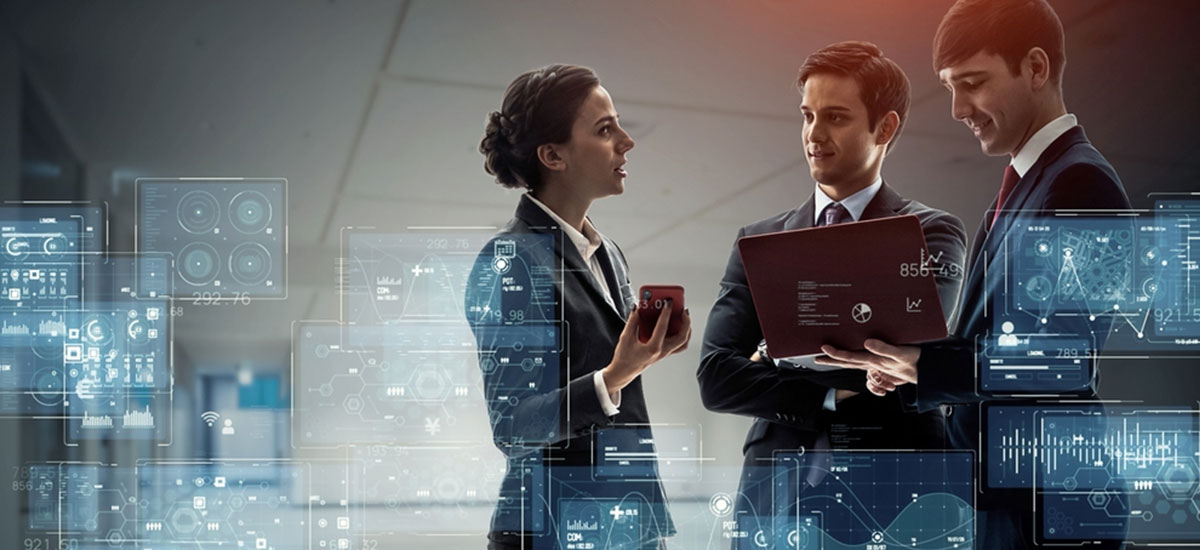
Listen to this blog
The advent of AI-powered forecasting was initially met with skepticism, with some businesses regarding it as nothing more than a gimmick to assert technological dominance over their competitors. However, as the need for organizations to optimize planning, achieve sustainable growth, and adapt to volatile market conditions continues to grow, AI-powered forecasting has become an indispensable tool.
Despite the significant investments made in these platforms, concerns persist over their reliability, which has resulted in a reluctance among some stakeholders to adopt these solutions.
Barriers to adopting AI-based forecasting
In our experience working with various clients, we have discovered that many of their concerns about AI-based forecasting are centered around the following factors:
⦁ Difficulty in interpreting forecast results in relation to business objectives.
⦁ The unexplainable impact of external factors on the forecast.
⦁ Overly technical platforms that require a steep learning curve.
⦁ Incompatibility of human intuition with the mathematical reasoning of AI models.
⦁ Monolithic platforms fail to address the unique needs of each business.
We will delve into each of these factors in more detail, but first, it's essential to understand what goes into creating better forecasting models that align with the specific requirements of businesses.
Building a business-centric forecast methodology
Creating forecasting models capable of handling specialized business needs is not an easy task. Data scientists must analyze the available data, identify any gaps, test the data quality, cater to any business-specific requirements, and suggest additional data for an optimal forecasting methodology. Developing an automatable, reproducible, and robust forecasting workflow is a challenging task. However, AI-powered forecasting can mitigate these challenges by providing sophisticated algorithms, standardized techniques, and big data handling capabilities. With AI, data scientists can create better models and develop fault-proof pipelines that can generate reproducible results, thus ensuring accuracy.
The forecasting conundrum: How can you trust what you can’t comprehend?
The use of AI-powered forecasting, while highly sophisticated, can present a challenge in terms of comprehensibility. For non-technical stakeholders such as business analysts, managers, and C-suite executives, the focus is on the implications of the predictions for their business, rather than the technical intricacies of the model. However, current AI platforms may not be equipped to provide sufficient rationale for the generated outputs, leaving users with unanswered questions and causing skepticism towards the reliability of the forecasts. Questions like “Why are certain values generated”?, What were the most significant contributing factors?” or "How do external factors impact the forecast?" remain unanswered, leading to reluctance in accepting the predictions without subjective modifications.
Strengthening trust in AI-driven forecasts
Establishing trust with users can be a challenging task when they have reservations about a proposed solution. As consultants or solution vendors, it is crucial not to assume that clients will invest their time to understand complex mathematical forecasting methodologies or adopt complicated workflows. To deliver maximum value and inspire confidence in the forecasting process, it is imperative to simplify and make the process as transparent as possible.
Features of a trust-worthy forecasting solution
To ensure the reliability and acceptance of our forecasting solutions, we have developed a trust model that serves as the cornerstone of our approach. By adhering to this model, we can generate accurate and trustworthy forecasts that win the trust of stakeholders.
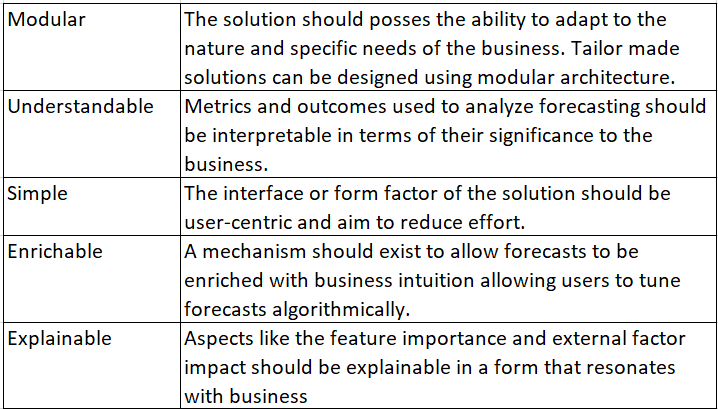
MUSEE Trust Model for Developing Forecasting Solutions
In the following sections, we will delve into the specific features that make a forecasting solution trustworthy, and how we integrate these features into our approach.
Strengthening Trust – by Ensuring Understandability
Clients' primary concern with forecasts is their inability to make sense of the predictions in terms of their business goals, financial KPIs, and strategic objectives. This is usually because forecast providers fail to convert or interpret these values into standardized business metrics that paint a clear picture of the parameters of interest for business users. To provide clients with easily understandable forecasts, it is important to ensure the following factors are guaranteed.
- Utilize a Forecast Interpreter
To ensure stakeholders can understand the forecasts, it's important for the forecast provider to have a solid understanding of the business objectives and dynamics. This will enable the provider to better explain the implications of the generated forecasts to stakeholders.
- Include Insightful Accuracy Metrics
While quantitative accuracy measures are important, they alone may not be sufficient for establishing stakeholder trust. Converting accuracy metrics into cost of decision metrics, such as forecast value add, overstocking expenses, and expected lost sales, can help stakeholders understand the business impact of the forecasts. Additionally, visual metrics such as baseline indicators, trend indicators, and growth rate bars can convey other important aspects of forecast health.
- Explain Results through “What-if” Scenarios
Complex relationships between inputs and outputs can sometimes make it difficult to understand isolated forecast results. Using what-if scenario-testing and comparing generated results can help stakeholders better understand how the model perceives different features and relationships within the data.
Strengthening Trust – by Providing Insight into External Factors
One way to enhance trust in forecasting solutions is by providing transparency into the impact of external factors on the forecasts. External datasets can provide valuable insights and improve the accuracy of forecasts. However, clients often want to know the individual influence of each factor on the forecasted results. For instance, a retail industry client may want to understand how the external factors being used are impacting the growth projections. To address this, we have developed a method that converts the impact of each external factor to dollar values for each time step. By doing so, we can clearly explain how each factor contributes to the overall forecast, allowing clients to better understand how the forecast aligns with their business goals and financial objectives.
Strengthening Trust – by Leveraging Business Insights to Improve Accuracy
Business users often express concerns about the objectivity of AI or mathematics-based models when it comes to forecasting. While these models can automate and streamline the result generation process, they may not take into account insights or nuances that could significantly impact future outcomes. To address this challenge, we have developed a standardized framework that leverages human intuition to enrich the generated forecasts without disrupting the patterns generated by the AI model. This approach helps to ensure that stakeholders have confidence in the accuracy of the forecasts, as they reflect the insights and intuitions they possess about the future.
Strengthening Trust – by Building a User-Centric and Adaptable Platform
The effectiveness of efforts to enhance forecast reliability can be undermined if users find the forecasting platform difficult to use or if a one-size-fits-all solution does not align with their business requirements. Therefore, it is crucial to develop forecasting platforms that are user-friendly and cater to varying levels of expertise. To accommodate the diverse needs of businesses, an ideal platform should have a modular architecture that allows customization of models, visualizations, and data solutions to suit specific business requirements.
Conclusion
The adoption of AI-based forecasting is crucial for businesses to stay competitive in the current market landscape. However, stakeholders are often wary of the technology due to limited technical expertise, complex infrastructures, and mistrust in the predictions generated. To overcome these challenges and establish trust in AI-generated forecasts, it is essential to develop user-friendly platforms and provide additional insights into forecast accuracy. Our MUSEE Trust Model has been designed to enhance the credibility, reliability, and usability of forecasting solutions. Overall, it provides a comprehensive approach to AI-based forecasting that addresses the challenges of limited technical know-how, complex solution infrastructures, and distrust in produced predictions. By implementing the MUSEE model, businesses can unlock the full potential of AI and gain stakeholder trust in the forecasts generated by the system.
Not sure where to start your AI-forecasting journey? Get in touch with Visionet today.