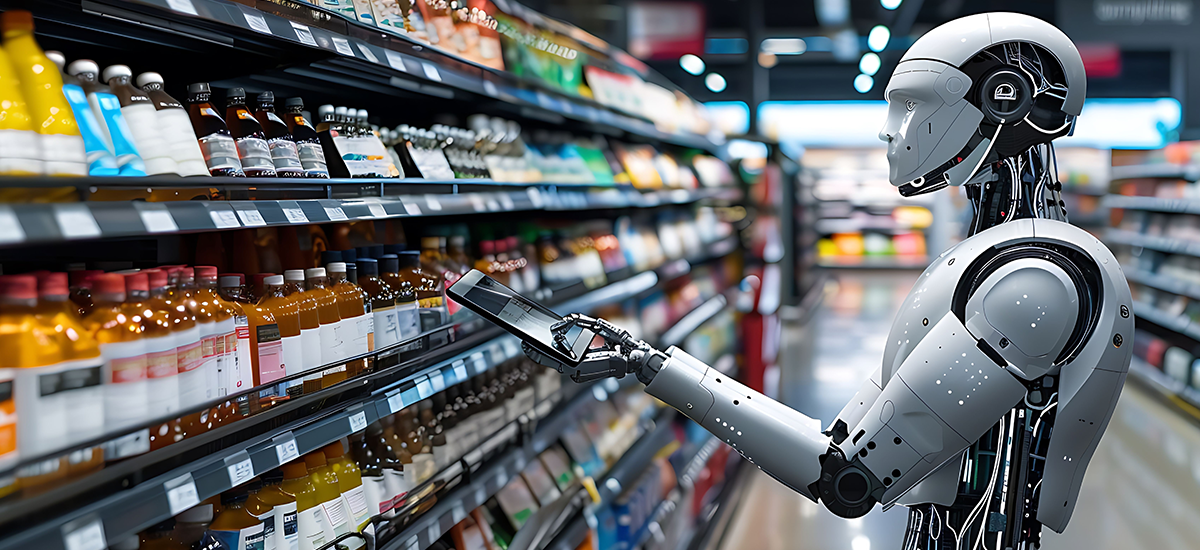
Listen to this blog
Introduction
Generative AI (GenAI) is revolutionizing the retail industry, offering new ways to personalize the shopping experience, optimize inventory, and streamline customer service. Statistics reveal that the Generative AI market in retail is projected to grow from $0.79 billion in 2024 to $1.11 billion in 2025, representing a compound annual growth rate (CAGR) of 39.8%. However, while pilots often demonstrate success in controlled environments, scaling GenAI models to full production can present significant challenges. These challenges include ensuring data quality, system integration, cost management, and a seamless transition from proof of concept to full-scale operations. In this blog, we’ll explore these common roadblocks and provide practical solutions to overcome them.
1. From test to reality: Closing the gap between retail AI pilots and full deployment
Challenge:
In retail, pilots often focus on isolated tasks like product recommendations or sales forecasting. However, when scaling these models, retailers face challenges like managing large datasets across various platforms, ensuring real-time processing, and maintaining compliance with industry regulations (such as GDPR). Technically, production environments may also deal with resource limitations, such as computing power or cloud capacity, that weren’t a concern during the pilot phase.
Solution:
Ensure that pilots are designed with scalability in mind. Adopt cloud infrastructure that can scale horizontally and accommodate real-time data processing. Leverage cloud services like AWS or Google Cloud to handle large amounts of transactional and customer data. Align AI initiatives with business objectives, like improving customer experience and optimizing inventory. Implement robust APIs to ensure seamless integration with existing retail systems, such as Point of Sale (POS) or inventory management systems.
2. Tackling data integrity: Ensuring consistency and compliance for retail AI solutions
Challenge:
Retailers often work with fragmented datasets coming from online and physical stores, social media, customer reviews, and product catalogs. Poor data quality and inconsistent governance can result in inaccurate AI predictions—like improper stock recommendations or flawed customer segmentation. Integrating and cleansing these datasets across multiple systems can become highly complex and resource-intensive.
Solution:
Implement a robust data governance framework that ensures data consistency across various systems. Use data lakes and platforms like Snowflake to centralize data storage, making it easier to clean, update, and manage. Leverage AI-driven data cleansing tools to improve the accuracy of the data. Regularly audit data quality and ensure that labeling is consistent across the entire pipeline to provide accurate information into the AI models. Additionally, consider privacy measures to comply with GDPR for consumer data.
3. Simplifying complexities: Managing the high costs and technical demands of AI in retail
Challenge:
Retailers may face high operational costs when scaling AI models. Fragmented AI tools often lead to inefficiencies, requiring different systems for data storage, analysis, and model training. The complexity increases as the volume of data grows, resulting in latency issues and rising costs for computing resources, especially for large-scale product recommendations or customer sentiment analysis.
Solution:
Adopt a unified AI platform to centralize all data storage and analysis processes. Cloud platforms like Snowflake or AWS enable retailers to manage their data and AI workflows efficiently, reducing redundancy and streamlining the entire pipeline. This helps reduce operational costs while improving AI performance by minimizing latency. Additionally, use auto-scaling features offered by cloud providers to scale resources as needed, ensuring a balance between performance and cost-efficiency.
4. Empowering retail teams: Making AI accessible and actionable for everyone
Challenge:
Technical complexity can prevent non-technical retail teams (marketing, customer service, etc.) from leveraging AI insights. A lack of training or communication between IT departments and business units can hinder the adoption of AI tools across the organization. This prevents valuable insights from reaching decision-makers, who need them to optimize customer engagement and marketing campaigns.
Solution:
To democratize AI access, invest in user-friendly and scalable AI platforms with intuitive interfaces that allow business users to easily run analytics and extract actionable insights. Use collaboration tools from platforms like Microsoft or AWS, which allow seamless communication between technical and business teams. Additionally, provide training to help non-technical staff understand how to interpret AI-driven insights and apply them to their everyday operations.
5. Navigating retail-specific regulatory hurdles and ethical considerations in AI
Challenge:
Retailers must comply with strict regulations (such as GDPR) that govern consumer data. Moreover, AI models may introduce biases that affect customer segmentation, pricing, or marketing strategies. Ensuring transparency in AI decision-making can be challenging, especially when using complex models like deep learning.
Solution:
Adopt AI governance frameworks that include encryption, anonymization, and compliance tools to secure customer data. Implement explainability techniques to make AI models more transparent, allowing stakeholders to understand how recommendations or decisions are made. Regularly audit AI models for bias and ensure that they comply with ethical guidelines and industry regulations.
6. Bringing GenAI into retail operations: Integrating AI seamlessly across processes
Challenge:
Integrating GenAI models into established retail workflows can be challenging, particularly when older systems (e.g., legacy POS systems, ERP software) need to work with modern AI tools. This integration is vital for minimizing disruptions in daily operations while maximizing the benefits of AI-driven decision-making.
Solution:
Ensure that AI models are designed to integrate seamlessly with existing systems through APIs and microservices. Use middleware platforms or integration tools to connect AI outputs with legacy systems, such as CRM, POS, and inventory management. Ensure that key stakeholders, from IT to business teams, are involved early to align AI projects with real business objectives.
7. Keeping retail AI in check: The importance of continuous monitoring and model maintenance
Challenge:
Retailers must ensure that AI models remain effective and relevant as customer preferences, seasonal trends, and market conditions evolve. Without continuous monitoring and model retraining, AI models can degrade over time, resulting in poor decision-making.
Solution:
Implement continuous monitoring tools that track key performance indicators (KPIs) such as sales conversions, customer satisfaction, and inventory turnover. Set up automated retraining pipelines using cloud-based machine learning platforms (e.g., AWS SageMaker, Google AI Platform) to keep models up-to-date. Use agile methodologies to iterate on models and quickly respond to changing market conditions.
8. Preparing retail organizations for change: Readiness for AI integration
Challenge:
Retailers may face resistance to adopting AI solutions due to concerns about job displacement, lack of AI literacy, or misalignment between business and IT teams. This organizational resistance can slow down the transition from pilot to full-scale deployment.
Solution:
Invest in change management programs that focus on educating employees about the benefits of AI. Provide training to increase AI literacy and emphasize that AI will complement, not replace, human decision-making. Ensure that business and IT teams align their objectives from the start, fostering a collaborative environment for successful AI implementation.
9. Evaluating the bottom line: Measuring retail AI’s ROI and managing costs effectively
Challenge:
Scaling AI in retail requires significant investment in infrastructure, software, and human resources. Measuring ROI can be difficult, especially when benefits like improved customer experience or inventory optimization are intangible or long-term.
Solution:
Define clear KPIs during the pilot phase to track improvements in areas such as customer engagement, sales performance, and inventory turnover. Use cloud-based pricing models (e.g., pay-as-you-go) to manage costs effectively, scaling resources based on real-time demand. Regularly assess the financial and operational impact of AI, adjusting strategies as necessary to ensure sustained ROI.
Conclusion
To succeed in scaling GenAI, retailers must prioritize integrating GenAI solutions into key customer-facing functions, from personalized marketing to inventory management, while ensuring compliance with data privacy regulations. They need to focus on creating seamless, AI-powered experiences that drive customer engagement and loyalty. Now is the time for retail leaders to embrace GenAI to stay competitive—explore how our GenAI services can transform your retail business today.